1D Kalman Filter
- Mun Kar Kin
- Jan 27, 2017
- 2 min read
Initially, ultrasonic sensors are used instead of the terarangerone sensors. Therefore, one can expect a significant drop in the quality. The filter is important to improve the consistency and the quality of the readings.
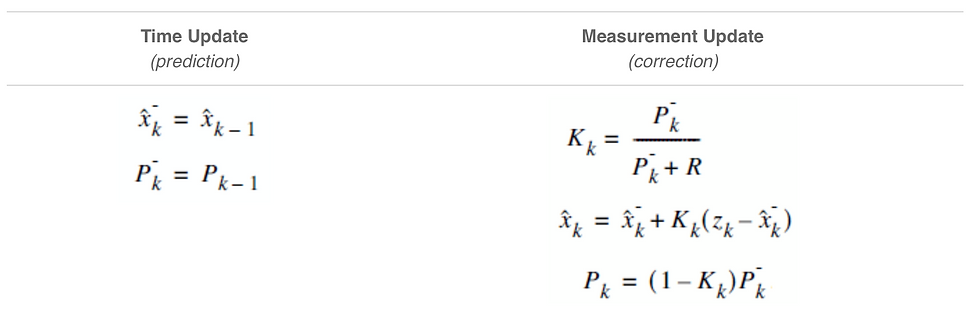
As indicated in the table above, there are two different distinct formulas, which are the Time Update and Measurement Update formulas, where:
𝑅 = 𝑁𝑜𝑖𝑠𝑒 𝑖𝑛 𝑡h𝑒 𝑒𝑛𝑣𝑖𝑟𝑜𝑛𝑚𝑒𝑛𝑡 𝑃 = 𝐸𝑠𝑡𝑖𝑚𝑎𝑡𝑒𝑑 𝐸𝑟𝑟𝑜𝑟 𝐾 = 𝐾𝑎𝑙𝑚𝑎𝑛 𝐺𝑎𝑖𝑛 𝑋 = 𝐹𝑖𝑙𝑡𝑒𝑟𝑒𝑑 𝐷𝑖𝑠𝑡𝑎𝑛𝑐𝑒𝑠 The formula works in iteration, and the accuracy of the formula is directly proportional to the number of
data points fed into the system.

By using a predetermined environmental noise value of R = 0.1, we have analysed the usefulness of the equations in Microsoft Excel. The distance of the sensor is set to be constant at 10cm away from the obstacle.


This formula is proven to be effective as can be seen in the two data representations above. For further analysis, other sets of data were obtained and analyzed.

At this stage, the formula is still working well to filter the values as the distance measured is constant. However, when the distance becomes a dynamic, problems began to arise.

The blue line represents the noise-filled values, while the orange line represents the filtered values. This is not acceptable as the Kalman filter treats the change in distance as noise. The reason behind this is because it is a 1-dimensional filter which is not appropriate for usage in this situation as distances of obstacles vary in real time.
The solution is either the use of Kalman Filter with more dimensions, or to use other filters such as a Low Pass Filter. Due to limited project time, a LPF is used instead.
Recent Posts
See AllWe have tested the image processing code on the Raspberry Pi and have found that performance is greatly impacted due to the Raspberry...
Comentários